Digital Twins: The New Frontier in Combining Complexity Science and Data Science to Tackle Global Challenges
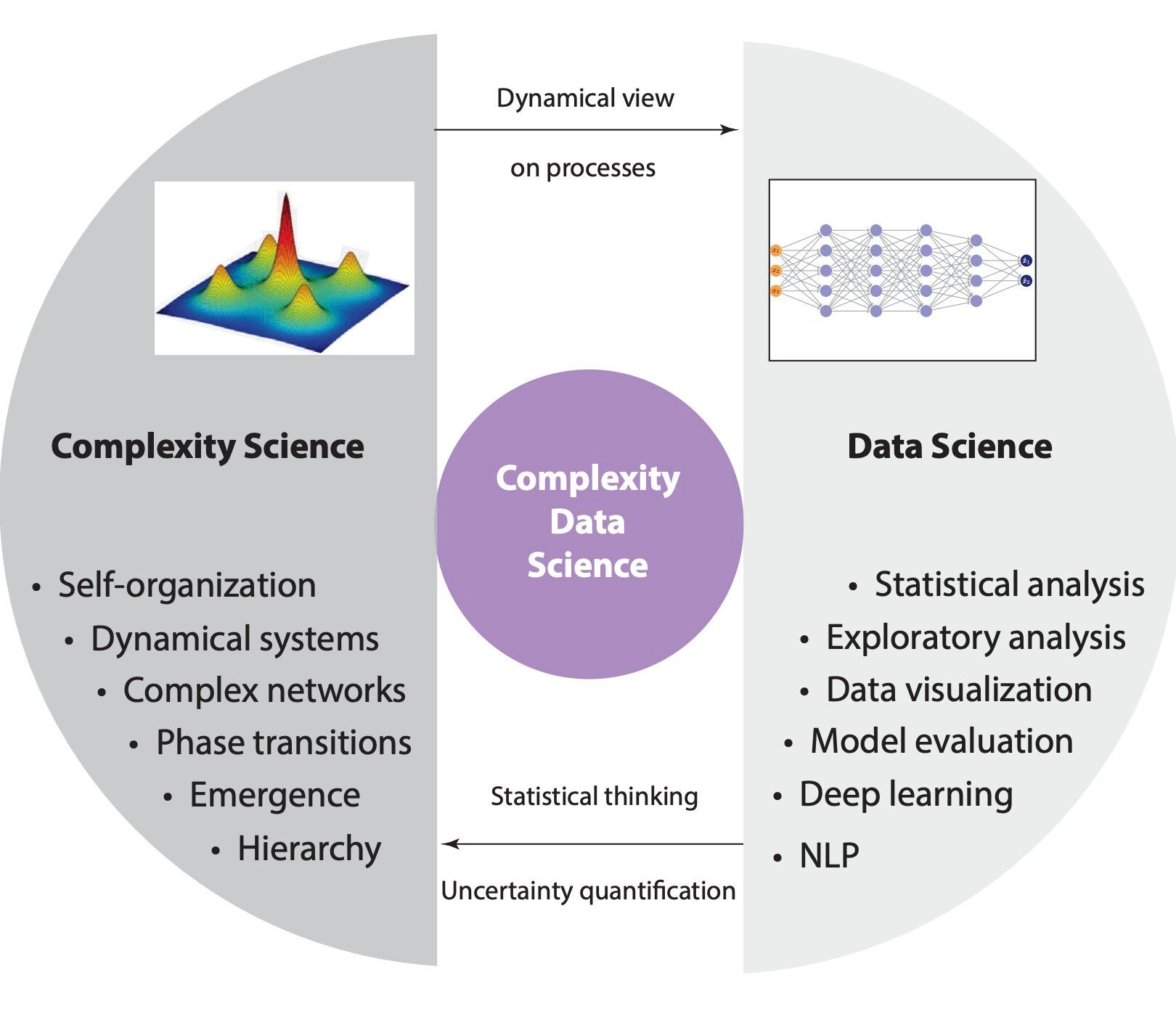
A groundbreaking perspective has emerged, suggesting that "digital twins" - a digital representation of real-world objects or systems - are the foundation for a new scientific field: complexity data science. Researchers Frank Emmert-Streib and colleagues argue that by integrating principles from complexity science and data science, researchers can gain unparalleled insights into complex systems, such as climate change and entire economies.
The concept of digital twins originated in manufacturing but has since been adopted by various fields, including medicine, immunology, and epidemiology. These digital replicas are typically continuously updated with real-time data from their physical counterparts, allowing for exploration of previously unexplored "what if" scenarios. The complexity of the simulated systems is a key link to complexity science, a field that studies complex phenomena in nature.
The study published in PNAS Nexus proposes the establishment of a new field: complexity data science. By combining techniques and insights from both complexity science and data science, researchers can tap into novel capabilities and provide innovative solutions to societal challenges. The development of explainable AI models, which provide transparency into decision-making processes, is seen as an exciting application of this new field.
As society grapples with pressing global issues, the potential of digital twins and complexity data science lies in their ability to unravel complex systems and offer transformative insights. Researchers are confident that this fusion of two scientific disciplines will pave the way for groundbreaking discoveries and tangible improvements in our daily lives.
The study, "Complexity data science: A spin-off from digital twins," is now available in PNAS Nexus with the DOI 10.1093/pnasnexus/pgae456.