AI Bridges the Gap Between Biological Tissue and Music, Redefining Materials Science
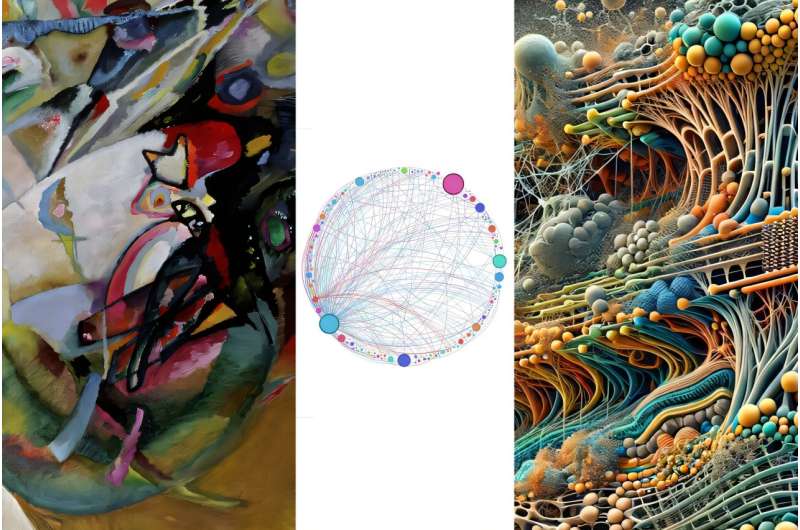
Boston, MA - A novel AI approach developed by Markus J. Buehler, professor of engineering and civil and environmental engineering at MIT, has found unexpected similarities between biological tissue and music, offering new insights into material design and innovation.
Using a graph-based AI model inspired by category theory, a branch of mathematics that deals with abstract structures and relationships, Buehler was able to analyze 1,000 scientific papers on biological materials and connect them in a knowledge map. The map revealed groups of related ideas and key points that link many concepts together.
In another experiment, the AI model recommended creating a new mycelium-based composite material inspired by an abstract painting by Wassily Kandinsky's "Composition VII". The result combines innovative concepts, such as a balance of chaos and order, adjustable property, porosity, mechanical strength, and complex patterned chemical functionality.
The AI approach uses graphs developed using methods inspired by category theory to teach the model to understand symbolic relationships in science. This framework enables the AI system to think about graph-based data, build better world representations models, and enhance its ability to explore new ideas to enable discovery.
Researchers can use this framework to answer complex questions, find gaps in current knowledge, suggest new designs for materials, and predict how materials might behave, and link concepts that had never been connected before. The application of this AI model could lead to the development of innovative sustainable building materials, biodegradable alternatives to plastics, wearable technology, and even biomedical devices.
"This study not only contributes to the field of bio-inspired materials and mechanics but also sets the stage for a future where interdisciplinary research powered by AI and knowledge graphs may become a tool of scientific and philosophical inquiry," Buehler said in a statement. "Graph-based generative AI achieves a far higher degree of novelty, explorative capacity, and technical detail than conventional approaches."
Buehler's work was recently published in Machine Learning: Science and Technology, an open-access journal that showcases cutting-edge research in machine learning and its applications.