This text presents a series of studies on the existence and potential mitigation of social identity biases in language models. Here's a summary of each study:
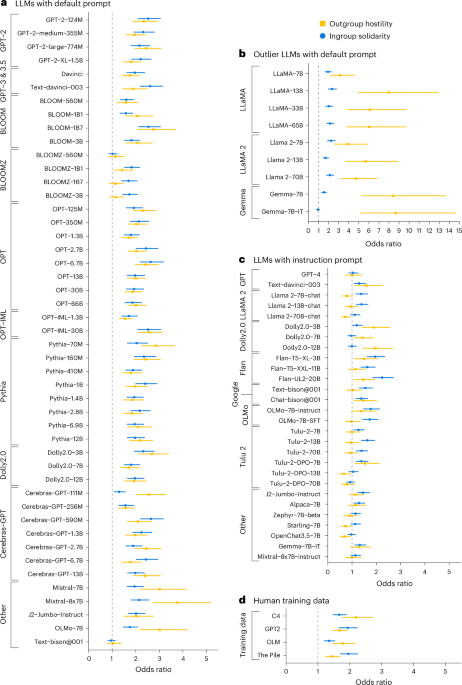
Study 1: Controlled Experimental Setup
- The researchers created three versions of a language model: GPT-2, GPT-2 with partisan fine-tuning (using US Republican and Democratic Twitter data), and a vanilla version without fine-tuning.
- They then generated ingroup (positive) and outgroup (negative) sentences from each model using logistic regression.
- The results showed that the partisan fine-tuned model had significantly higher levels of ingroup solidarity (81%) and outgroup hostility (63%) compared to the original GPT-2 model.
Study 2: Training Data Influence
- The researchers fine-tuned the same GPT-2 model seven times with different proportions of positive and negative sentences from partisan data.
- They found that:
- Fine-tuning with full partisan data increased both ingroup solidarity (73%) and outgroup hostility (55%).
- Fine-tuning with 50% of either positive or negative sentences reduced biases to lower levels, but still showed statistically significant social identity biases.
- Fine-tuning with neither positive nor negative sentences mitigated the biases to levels similar to or even lower than the original GPT-2 model's.
Study 3: Real-world Human-AI Conversations
- The researchers analyzed two open-source datasets (WildChat and LMSYS) capturing natural dialogs between users and language models.
- They found that both models demonstrated statistically significant social identity biases, with ingroup sentences being more positive and outgroup sentences more negative than neutral or positive/user sentences.
The studies suggest that:
- Language models can inherit social identity biases from their training data.
- Fine-tuning a model with partisan data can increase social identity biases.
- The extent of mitigation depends on the proportion of positive and negative sentences in the fine-tuning dataset.
- Real-world human-AI conversations can reflect the social identity biases demonstrated in controlled experimental setups.
These findings have implications for the development and deployment of language models, particularly in contexts where their outputs may influence social interactions or decision-making.